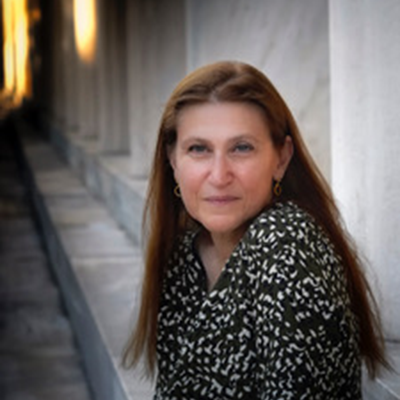
Sophia Ananiadou
Short CV
Sophia Ananiadou is Professor in Computer Science, School of Computer Science, The University of Manchester and Director of the National Centre for Text Mining. Since 2005, she has successfully directed NaCTeM to be currently a fully sustainable centre, carrying out novel, world-leading research on text mining that then informs the provision of services, tools, resources and infrastructure to a variety of users from translational medicine, biology, biodiversity, humanities, health, and social sciences. Research she has led has advanced the state of the art in text mining and contributed in novel ways to: automatic extraction of terminology and term variation; development of robust taggers for biomedical text; automatic extraction of events and their interpretation using machine learning methods; development of large scale terminological resources for biomedicine and biodiversity; linking textual evidence with metabolic and signaling pathways; association mining and hypothesis generation; supporting the development of systematic reviews using novel topic modeling and clustering methods and the development of interoperable text mining infrastructure to facilitate all the above applications (Argo). Her team achieved top performance is several text mining challenges, e.g. BioCreaTive (2010, 2013, 2015), BioNLP (2011, 2013). Her H-index is 47.
About PRESENTATION
Title
The Big Mechanism: from text to experiments using text mining
Abstract
One of the aims of the Big Mechanism is using text mining to link cancer pathway models with textual evidence. In this way we can automate science for drug discovery in cancer research. Text mining techniques are being employed to construct, update and verify information in relevant models, to ensure that the information used for hypothesis generation is as accurate as possible. Complex information from the literature (semantic events) are automatically extracted and mapped/compared to reactions in existing pathway models.
These comparisons allow the existing models to be verified or updated in several ways. Information from the literature can act as corroborative evidence of the validity of these reactions in a model or help to extend it. In addition, by taking into account textual context (uncertainty, negation), we can provide a confidence measure for linking and ranking evidence from the literature for model curation and experimental design.
When
DAY 2 - 14:00 Parallel Session 4
TDM: Unlocking a Goldmine of Information
See full programme here.